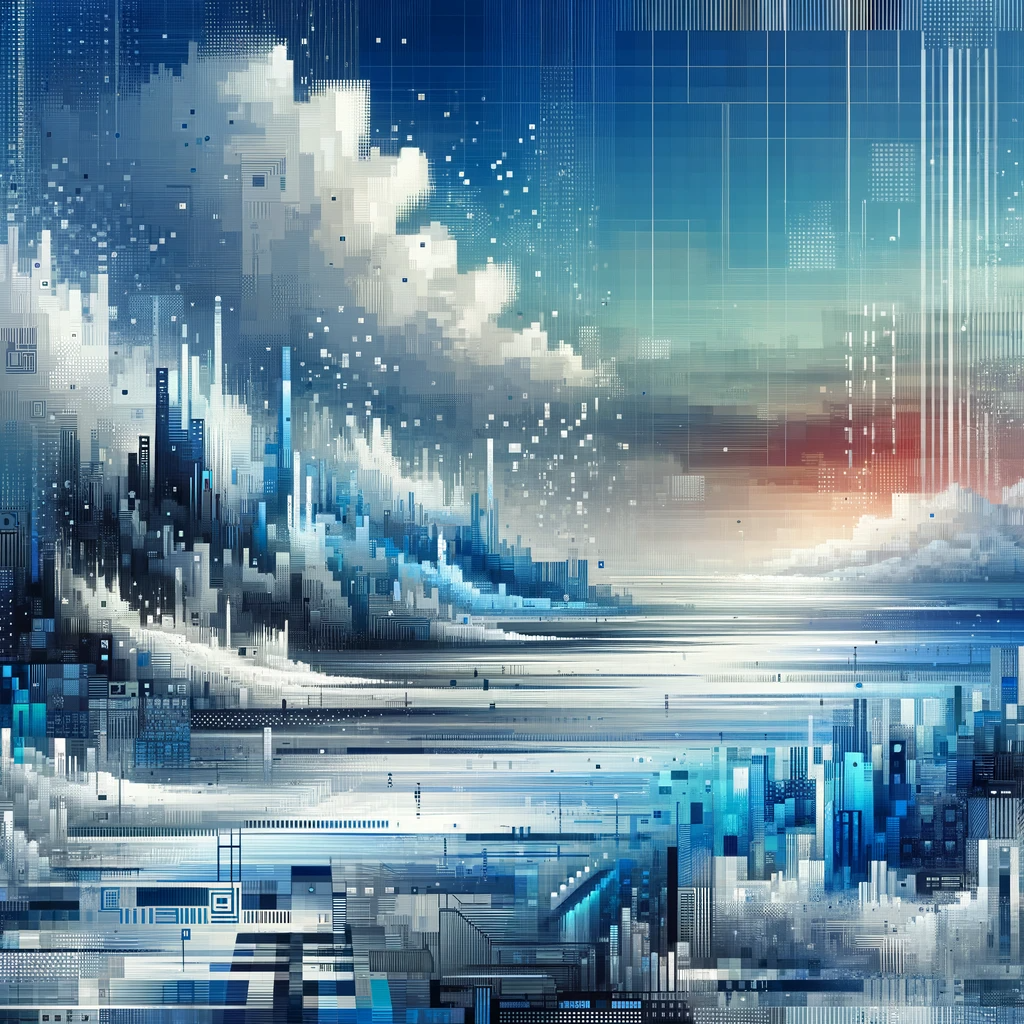
Is Winter Coming? —A Shift Towards a More Realistic View of the AI’s (Near) Future
In the mainstream world of technology, during 2023, there were few challengers to Generative Artificial Intelligence (GAI). Expectations are now undoubtedly heightened. The question now is whether the big tech companies will be able to live up to unprecedented expectations, or will the inevitable disillusionment set in. And if so, what will be left of this currently shining industry?
Up until the end of 2023, most opinions tended to follow a more jubilant optimistic line, but since then a sharp divergence has also emerged. Elon Musk, for example, has argued that AI could even bring a world in which human labor is no longer needed. For this reason, he believes that economies need to start preparing for the special taxes that will be levied on specific industries, especially the tech sector. At the same time, others are predicting the next winter of AI as early as 2024, a period during which investment in the sector will dry up and interest in the technology will fall sharply.
A similar trend reversal would not be unprecedented, as it happened in the 1970s. At the time, this was mainly because of unfulfilled promises. The dominant trend of the time, machine translation, promised to enable, for instance, Russian texts to be translated into English, with virtually no human intervention. In the Cold War political climate of the time in the US, of course, this raised huge expectations and attracted significant investment. When, years later, such attempts clearly failed, it brought investor disillusionment.
The situation now is different in that in the 1960s and 1970s, AI development was mainly funded by government money. Today, on the other hand, the technology is pioneered by market-based companies whose value is significantly influenced, for example, by the price of their shares on the stock exchange.[1] This is why perhaps the most important question about the near future of AI today is the ability of development companies to generate profits for their shareholders.
Since its launch, the GAI has undoubtedly been the subject of considerable hype. The headlines on AI in recent years have focused mainly on the developments of big tech companies, the transformative importance of AI, and the impact of new technologies on everyday life. But less has been said about how these developments will be put into practice, and how the mega projects of mega corporations will become widely adaptable technologies. How smaller players in the market can make them profitable for themselves and their users was also neglected.
The latter is particularly relevant, given that anyone who wants to produce state-of-the-art quality today has basically three options.
- One can sign a contract with a tech giant and sub-license their own solution based on development under a specific contract.
- Own solutions can be developed. To be competitive in today’s market, it is most practical to rely on open-source solutions, also developed by large companies. An interesting issue here is the original license, which either allows (with certain restrictions) or prohibits any use for profit.
- One may be able to develop a standalone solution (e.g. custom LLM training), but this will have a significant cost (up to the order of millions of dollars).
In addition to the above, profitability also depends on what a given model, application, etc. will be able to do in a real use case (not just a use case built for a demonstration of power). The example of ChatGPT shows that industrial adaptation is still in the pathfinding phase. In fact, Bard, ChatGPT, or specialized services based on them (e.g. GitHub Copilot) already provide interesting and often useful services today. However, considering that some estimates put the cost of running ChatGPT at hundreds of thousands of dollars in a single day, it may still be a serious problem to develop usage-based or subscription-based models that are acceptable to users and operators as well.
Perhaps it is the reason why concerns and often controversial news are emerging about whether AI is one of the biggest bubbles of our time. The tech sector has never lacked for such things, just think of the dotcom bubble, which is often compared to the current situation. But so was the huge stock market boom before cryptos and the NFT crash.
It is almost certain that some kind of consolidation in the AI market is slowly becoming inevitable. There are predictions that a total collapse is likely, and the current state of AI is clearly described as a bubble. The main question here is what can be “recycled” from the AI developments that are falling apart. I think it is very likely that such a bubble has developed in the last years indeed. But where it goes from here, once it bursts, will be determined more by whether the general enthusiasm can be sustained until the GAI applications are able to trickle down from the tech giants to at least mid-sized companies. There are already signs of this trend, although progress in this direction is not rapid.
On the cost reduction side, new technologies may be emerging, such as quantization, which can reduce the size of models, or federated learning, which can save on the production of training data. Another promising technique could be prompt compression, which saves the user a usage-based fee and the operator runtime by compressing the information sent to the Large Language Models. But almost all such innovations are still in their infancy.
In the case of non-generative AI, we see that there are now abundant areas where supervised Machine Learning algorithms, such as clustering algorithms, are applied routinely and with high confidence (just think of LegalTech tools). The most likely scenario is that GAI will follow this path soon and based on current trends, I believe it can go this way without experiencing another “long AI winter”.
[1] OpenAI is an exception, given that the company has not (yet) been listed on the stock exchange.
István ÜVEGES is a researcher in Computer Linguistics at MONTANA Knowledge Management Ltd. and a researcher at the HUN-REN Centre for Social Sciences, Political and Legal Text Mining and Artificial Intelligence Laboratory (poltextLAB). His main interests include practical applications of Automation, Artificial Intelligence (Machine Learning), Legal Language (legalese) studies and the Plain Language Movement.